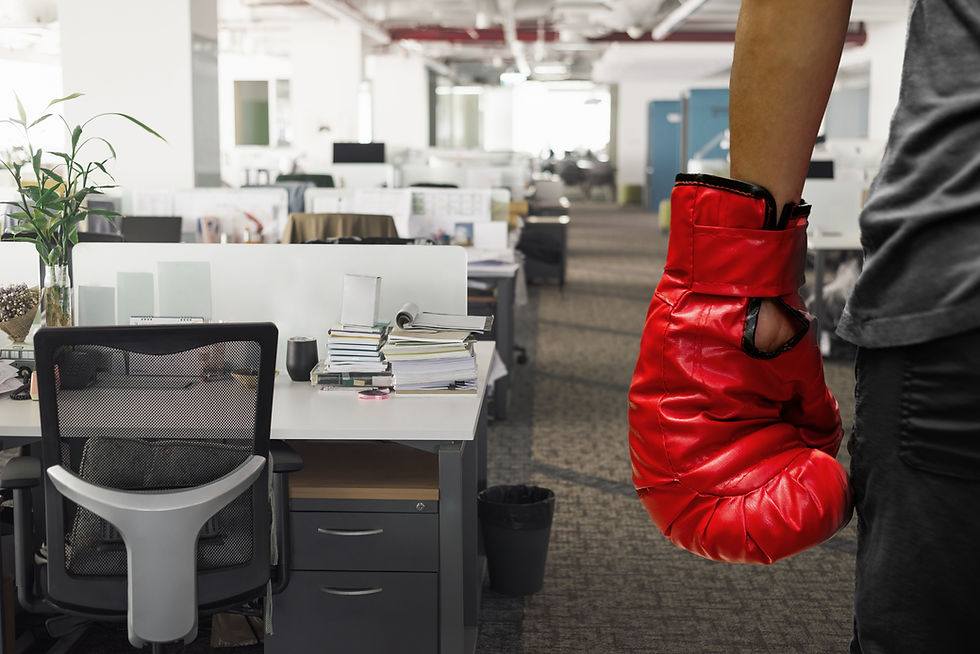
Make no mistake about it. Data is a critical business asset. Therefore, control of data is power. Where there is power and control, politics will follow.
The control of data and insights within an organization will not necessarily play out as power struggles and political battles - with the result being one person or team ending up the "winner" and the other the "loser". But it certainly can - and along the way, the organization as a whole may end up in the" loser" column.
However, it must be noted that the natural tensions and dynamics of people coming together to accomplish something - in this case the generation and consumption of data - can be healthy when managed properly. When done with the best interest of the organization rather than any individual or group, constructive challenges to how data is managed can be beneficial in ensuring data - as a corporate asset - is being utilized to its full potential.
As every organization is different - with unique personalities and culture for dealing with conflict - we won't be delving into the "how to deal with (and win!) the politics of data" in this article. Such an endeavor would require an entire book to properly tackle.
Instead we will outline the various organizational dynamics that can contribute to unnecessary power battles in the data domain.
Awareness of these dynamics will be beneficial for all parties - those who manage the data, those who generate insights, those who leverage the insights to drive the business, and leadership - in 1) helping prevent data politics from forming in the first place, and 2) dealing with it if it starts happening.
The data landscape contains several places where the struggle for control may surface - and the resulting political tensions would not be far behind:
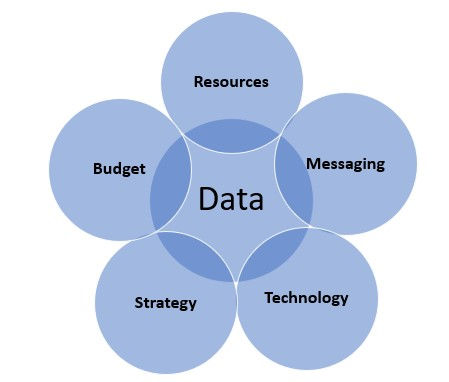
Let's dig a bit deeper into each of these areas....
Control of the Data
The scope of "Data" for our purposes here is the actual data: the data sets / subject areas, business logic and transformation, its physical storage, as well as the metrics generated from the data sets.
In most organizations the data owners are the related lines of business - for example, sales forecasts, the opportunity pipeline, quotas, etc. are owned by the sales org. The data owners may also have the governance roles of data trustees and data stewards (who are formally responsible for the source data sets and any metrics that come from this source data).
So, if we have data owners/trustees/stewards - where are the seeds of data politics sewn?
Probably the most common battle for control of the data is when business functions are controlling the access to "their" data and/or withholding details (business logic and calculations) for "their" metrics.
The politics come about primarily because those who control the data (and metrics) are also often the ones who get to control the narrative around the numbers.
This often plays out when a functional business leader trusts a particular data set, database, and team to provide their metrics and insights.... and these live outside the "mainstream" analytics.
While not usually nefarious, these situations are often borne from the early, informal days of the company analytics activities... when everyone was forced to do whatever was necessary to get their insights.
Over time, most business functions will give up this control (of the storage of data and the exclusive ability to analyze the data) in exchange for having a properly managed analytics environment and access to the rest of the corporate data. However, some business functions will consider this abdication of control too risky.
The refusal to give up control is often accompanied by:
Unnecessarily rigid control of access to data beyond legal, privacy, security, or confidentiality reasons.
Data silos that remain in place after the development of a central data warehouse.
Lack of visibility to the business logic - the "black magic" of how metrics are calculated.
These types of data controls have several negative consequences:
increased cost in maintaining multiple data platforms
confusion caused by having multiple "sources of truth" for reporting and analytics
opportunity cost of not having the data available for cross-functional analytics
Prevention (if caught early) or minimizing the impact of political control over data can include establishing the following:
a culture of data democratization ("the data belongs to the company, not any one group")
common processes and workflows for data access requests and provisioning
a formal process for identifying and retiring redundant data silos
a data catalog and glossary which exposes the underlying data and logic to enable anyone with the proper access to generate metrics
Control of the Analytics Resources (people)
With control of the people performing the various analytics functions (data engineering, data analysis, data science, governance, stakeholder engagement) comes the ability to control:
roles and responsibilities
hiring decisions
what projects get approved
prioritization of projects
timelines
Improperly managed analytics resources will likely lead to "stealth politics" - the warning signs of which will may include:
the hiring of redundant data resources by the business functions
the creation of "shadow" or unofficial analytics efforts
proliferation of rogue databases and technology stacks
The above almost always occur because the business functions are not getting what they need from the analytics function - often in form of less-than-satisfactory turn-around times for key analytics initiatives.
The end result is an unnecessary increase in operating costs, and confusion for the organization caused by multiple sources of truth (systems) and analytics.
Prevention - or at least mitigating - the politics of analytics resources may be possible through the following efforts:
Reducing the resource dependency by enabling (more) analytics "self-service" capabilities.
Revamping the org structure where the analytics resources currently reside - such as implementing a matrix reporting structure.
Re-engineering the project (resource) prioritization process to enable more flexibility in the roadmap.
Strengthening the collaboration between the analytics resources and the business functions - such as bringing the stakeholders into the development and deployment process earlier in the cycle (instead of at the very end during UAT).
Control of the Analytics Technology
This is the one area where there should not be any question about control of the foundational analytics technology. It most certainly belongs with the same organization that controls the other core business systems. Defining the integrated architecture of all business systems and the underlying data models belongs in the IT space.
There is however at least one technology-related political issue that organization will have to face...
Who controls the "non-core" analytics applications?
Business functions often need data-related functionality above and beyond what is provided by the "core" analytics systems/tools/applications: data warehouse, ETL tools, data viz tools, etc.
The question will arise at some point of how much control the technology team has in regards to deciding on and approving the use of "non-core" analytics applications. Should the business function have autonomy in deciding on and procuring, for example, a data governance tool or a tool to manage analytics projects?
The business function will almost always argue in favor of autonomy. As these applications are typically stand-alone, the technology team will often be OK with letting the business control the decision and procurement process - though usually only after a review process to ensure the application will not impact the infrastructure.
However, things get much murkier when you are talking about analytics applications that touch or directly access data from IT-managed systems. For example, what about self-service data aggregation tools such as Alteryx? Or, data science tools? Or a few licenses to try out a new data viz tool?
Even if the application will be funded by the business function out of its own budget, the technology team will rightly have concerns about application / tool proliferation. Oh, and for that matter, so your procurement team (if one exists) will also care about application proliferation as well as unnecessary and costly redundancy.
And generally speaking, these concerns are valid. No analytics function will reach its full potential if there are a half-dozen data visualization tools in production.
So how does an organization deal with the politics of control over these "non-core" analytics technologies (or even determine which technologies are considered "core")?
Well, for organizations that have a robust analytics strategy, the high-level guidelines are likely already in place. Are "business driven" and "self-service" central themes? Then non-core applications that support this strategy would likely be under the control (final decision on which application) of the business selves being serviced.
For organizations where such distinctions have not already been made?
Well, as advocates for "business driven self-service analytics", here is how we approach this:
"If the application does not require any integration (coding) with other business systems, has minimal data/network bandwidth requirements, and there are no security concerns, the decision and ownership can remain with the business.
However, any time the application requires integration, significant infrastructure resources, or has any security considerations, it should be under the purview of the technology team."
Either way, to prevent future political battles down the road, now would be a good time to hash it out and add this piece to your current analytics strategy (which does exist, right?)
Control of the Analytics Messaging
Who controls the messaging of what is being done in analytics and socializing the accomplishments?
As analytics initiatives become larger they will involve multiple teams across the organization. This also means these activities will have higher visibility. With that visibility comes the politics of "who gets credit for delivering the analytics?". THIS is where the politics of messaging come into play.
Does credit go to the data engineering team who architected the technical solution, brought the data into the warehouse, and integrated it into the data model so that it can be consumed by the business?
Does it go to the analytics team that took the data and developed insights for the business?
Does it go to the business teams that took the insights and made the necessary decisions and took the action to improve the business?
Of course, the answer is "yes" to all of the above - as without the other two functions, the organization as a whole would not be able to turn data into business transformation.
Before proceeding - now is a good time to touch on an important side note:
Beware the technology team being left-out of the messaging. Rarely is this by design or intent, but it does happen as the business-facing functions are providing messaging on analytics activities to their leadership - where the accomplishments of the business team overshadow those of the technology team. This is quite common and is often the unspoken/unrecognized source of many political battles between technology teams and business facing analytics teams.
So, while all parties in the analytics supply chain deserve credit - there is still the question of who controls the messaging:
Who sends out the "Congratulations on going live with the new predictive model" email to senior leadership?
Who gets the face-time at the executive QBR?
Who gets on stage at the company all-hands?
Again, there are no universal right answers (other than the messaging should be jointly crafted and delivered), but this is an area to consciously consider and address to head off political battles and the ensuing, and often buried, resentment.
Control of the Analytics Strategy
Fundamentally, an organization must decide if the analytics function should be business driven or technology driven.
Analytics are considered business-driven when the business functions (as a group) control the following:
Analytics Strategy
Project Prioritization
Project Management
Stakeholder Engagement
Non-core analytics tools
Analytics budget
Technology-driven analytics is when the technology team controls these areas.
Politics come into play in this space quite frequently as in many ways it can be viewed as an overlay of the control of the budget, resources, and organization structure.
Consider for a moment the situation where the team that controls the analytics budget is not the one in control of the analytics strategy.
That is just begging for a political battle to break out.
The "technology-driven vs business-driven" decision needs to be made not only a part of the analytics strategy, but should also be reflected in the organization structure.
A decision for business-driven analytics should result in the formation an "Enterprise Analytics" organization that reports under the COO or a line of business "C"-level.
A decision for technology-driven analytics will have the functions related to strategy, prioritization, and stakeholder engagement under the CIO.
Neither approach is fundamentally right or wrong, but there needs to be a clear, conscious, and well-communicated decision at the executive leadership level as to which approach the organization is to be aligned.
Control of the Analytics Budget
Money. The ultimate in control and power. The ultimate in politics. Just look at the US Congress.... Pandemic relief packages. Health coverage.
Thankfully, the politics of your analytics budget won't end up being broadcast on C-SPAN.
It can however be contentious - and have significant ramifications if not handled well.
First and foremost is where the analytics budget comes from and who controls it. This is usually well-defined in most organizations, but as the analytics function grows, it needs to be revisited.
As such, at some point, it WILL become political.
Consider for a moment an analytics organization that has 5 people. This team is not likely to need its own budget.
Now on the opposite end of the scale... an analytics function with 500 people. They will likely need their own budget.
Somewhere in between is the inflection point. The good news is that this will eventually play itself out when the organization brings on board a dedicated VP of analytics. The bad news is that until that point in time, there will likely be mini battles that the organization will be forced to deal with.
The second budget-related political area is the budget process.
This is fraught with many landmines. So, keep a pulse on how well the budget process plays out:
Who manages the overall process
Who else is involved in the process
What is the scope (applications? infrastructure? people?)
What are the processes for:
- Requests
- Prioritization
- Arbitration
- Allocation
- Final decision
Each of the above areas are subject to political battles...
So, careful and diligent management of the process should strive to ensure the following:
Is there fair representation in the decision process?
If there are budget allocations, are they fair?
Are there provisions for "business funding" if projects are not approved in the overall analytics budget?
Even with careful and diligent management, there will be "winners" and "losers" in the budget process. Acknowledge this with all parties and be prepared to manage the fallout - as this is one of the root causes of "shadow" analytics teams and rogue siloed databases.
So while there are few if any concrete ways to prevent politics in the data realm, you are now armed with insights into where - and why - the myriad of political battles can form.
With this awareness you will be in better position to get ahead of the curve - and help:
build out a culture of data democratization
create a healthy analytics organizational structure
build an analytics strategy that includes clearly defined ownership of key analytics elements
ensure fair and complete messaging of analytics accomplishments
We'd like to leave you with one more thing to ponder - something perhaps a bit too deep to address in a (relatively) short article:
The potential for significant political battles is high if there is a mis-alignment of analytics roles / responsibilities, reporting structures, and the perceptions and actions of the organization.
Comments